In
mathematics, specifically
statistics and
information geometry
Information geometry is an interdisciplinary field that applies the techniques of differential geometry to study probability theory and statistics. It studies statistical manifolds, which are Riemannian manifolds whose points correspond to p ...
, a Bregman divergence or Bregman distance is a measure of difference between two points, defined in terms of a strictly
convex function
In mathematics, a real-valued function is called convex if the line segment between any two points on the graph of the function lies above the graph between the two points. Equivalently, a function is convex if its epigraph (the set of poin ...
; they form an important class of
divergence
In vector calculus, divergence is a vector operator that operates on a vector field, producing a scalar field giving the quantity of the vector field's source at each point. More technically, the divergence represents the volume density of t ...
s. When the points are interpreted as
probability distribution
In probability theory and statistics, a probability distribution is the mathematical function that gives the probabilities of occurrence of different possible outcomes for an experiment. It is a mathematical description of a random phenomeno ...
s – notably as either values of the parameter of a
parametric model
In statistics, a parametric model or parametric family or finite-dimensional model is a particular class of statistical models. Specifically, a parametric model is a family of probability distributions that has a finite number of parameters.
Def ...
or as a data set of observed values – the resulting distance is a
statistical distance
In statistics, probability theory, and information theory, a statistical distance quantifies the distance between two statistical objects, which can be two random variables, or two probability distributions or samples, or the distance can be b ...
. The most basic Bregman divergence is the
squared Euclidean distance
In mathematics, the Euclidean distance between two points in Euclidean space is the length of a line segment between the two points.
It can be calculated from the Cartesian coordinates of the points using the Pythagorean theorem, therefore o ...
.
Bregman divergences are similar to
metric
Metric or metrical may refer to:
* Metric system, an internationally adopted decimal system of measurement
* An adjective indicating relation to measurement in general, or a noun describing a specific type of measurement
Mathematics
In mathem ...
s, but satisfy neither the
triangle inequality
In mathematics, the triangle inequality states that for any triangle, the sum of the lengths of any two sides must be greater than or equal to the length of the remaining side.
This statement permits the inclusion of degenerate triangles, bu ...
(ever) nor symmetry (in general). However, they satisfy a generalization of the
Pythagorean theorem
In mathematics, the Pythagorean theorem or Pythagoras' theorem is a fundamental relation in Euclidean geometry between the three sides of a right triangle. It states that the area of the square whose side is the hypotenuse (the side opposit ...
, and in information geometry the corresponding
statistical manifold is interpreted as a (dually)
flat manifold In mathematics, a Riemannian manifold is said to be flat if its Riemann curvature tensor is everywhere zero. Intuitively, a flat manifold is one that "locally looks like" Euclidean space in terms of distances and angles, e.g. the interior angles of ...
. This allows many techniques of
optimization theory
Mathematical optimization (alternatively spelled ''optimisation'') or mathematical programming is the selection of a best element, with regard to some criterion, from some set of available alternatives. It is generally divided into two subfi ...
to be generalized to Bregman divergences, geometrically as generalizations of
least squares
The method of least squares is a standard approach in regression analysis to approximate the solution of overdetermined systems (sets of equations in which there are more equations than unknowns) by minimizing the sum of the squares of the r ...
.
Bregman divergences are named after Russian mathematician
Lev M. Bregman
Lev M. Bregman (1941 - 2023) is a Soviet and Israeli mathematician, most known for the Bregman divergence named after him.
Bregman received his M. Sc. in mathematics in 1963 at Leningrad University and his Ph.D. in mathematics in 1966 at the ...
, who introduced the concept in 1967.
Definition
Let
be a continuously-differentiable, strictly
convex function
In mathematics, a real-valued function is called convex if the line segment between any two points on the graph of the function lies above the graph between the two points. Equivalently, a function is convex if its epigraph (the set of poin ...
defined on a
convex set
In geometry, a subset of a Euclidean space, or more generally an affine space over the reals, is convex if, given any two points in the subset, the subset contains the whole line segment that joins them. Equivalently, a convex set or a convex ...
.
The Bregman distance associated with ''F'' for points
is the difference between the value of ''F'' at point ''p'' and the value of the first-order
Taylor expansion
In mathematics, the Taylor series or Taylor expansion of a function is an infinite sum of terms that are expressed in terms of the function's derivatives at a single point. For most common functions, the function and the sum of its Taylor se ...
of ''F'' around point ''q'' evaluated at point ''p'':
:
Properties
* Non-negativity:
for all p, q. This is a consequence of the convexity of F.
* Positivity: When F is strictly convex,
iff
.
* Uniqueness up to affine difference:
iff
is an affine function.
* Convexity:
is convex in its first argument, but not necessarily in the second argument. If F is strictly convex, then
is strictly convex in its first argument.
** For example, Take f(x) = , x, , smooth it at 0, then take
, then
.
* Linearity: If we think of the Bregman distance as an operator on the function ''F'', then it is linear with respect to non-negative coefficients. In other words, for
strictly convex and differentiable, and
,
::
* Duality: If F is strictly convex, then the function F has a
convex conjugate
In mathematics and mathematical optimization, the convex conjugate of a function is a generalization of the Legendre transformation which applies to non-convex functions. It is also known as Legendre–Fenchel transformation, Fenchel transformatio ...
which is also strictly convex and continuously differentiable on some convex set
. The Bregman distance defined with respect to
is dual to
as
::
:Here,
and
are the dual points corresponding to p and q.
* Mean as minimizer: A key result about Bregman divergences is that, given a random vector, the mean vector minimizes the expected Bregman divergence from the random vector. This result generalizes the textbook result that the mean of a set minimizes total squared error to elements in the set. This result was proved for the vector case by (Banerjee et al. 2005), and extended to the case of functions/distributions by (Frigyik et al. 2008). This result is important because it further justifies using a mean as a representative of a random set, particularly in Bayesian estimation.
* Bregman balls are bounded, and compact if X is closed: Define Bregman ball centered at x with radius r by
. When
is finite dimensional,
, if
is in the relative interior of
, or if
is locally closed at
(that is, there exists a closed ball
centered at
, such that
is closed), then
is bounded for all
. If
is closed, then
is compact for all
.
* Law of cosines:
[https://www.cs.utexas.edu/users/inderjit/Talks/bregtut.pdf ]
For any
::
*
Parallelogram law
In mathematics, the simplest form of the parallelogram law (also called the parallelogram identity) belongs to elementary geometry. It states that the sum of the squares of the lengths of the four sides of a parallelogram equals the sum of the ...
: for any
,
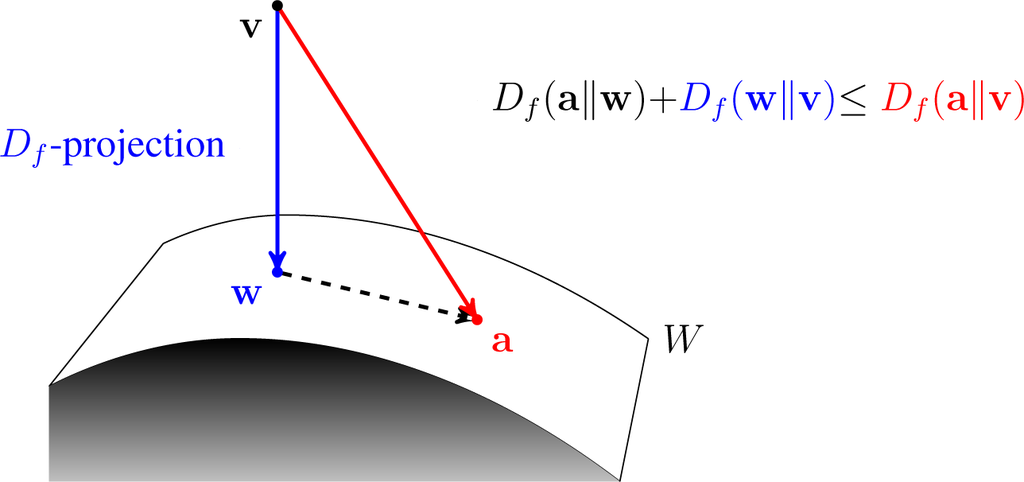
* Bregman projection: For any
, define the "Bregman projection" of
onto
:
. Then
** if
is convex, then the projection is unique if it exists;
** if
is closed and convex, and
is finite-dimensional, then the projection exists and is unique.
* Generalized Pythagorean Theorem:
For any
,
This is an equality if
is in the
relative interior
In mathematics, the relative interior of a set is a refinement of the concept of the interior, which is often more useful when dealing with low-dimensional sets placed in higher-dimensional spaces.
Formally, the relative interior of a set S (deno ...
of
.
In particular, this always happens when
is an affine set.
* ''Lack'' of triangle inequality: Since the Bregman divergence is essentially a generalization of squared Euclidean distance, there is no triangle inequality. Indeed,
, which may be positive or negative.
Proofs
* Non-negativity and positivity: use
Jensen's inequality
In mathematics, Jensen's inequality, named after the Danish mathematician Johan Jensen, relates the value of a convex function of an integral to the integral of the convex function. It was proved by Jensen in 1906, building on an earlier ...
.
* Uniqueness up to affine difference: Fix some
, then for any other
, we have by definition
.
* Convexity in the first argument: by definition, and use convexity of F. Same for strict convexity.
* Linearity in F, law of cosines, parallelogram law: by definition.
* Duality: See figure 1 of.
* Bregman balls are bounded, and compact if X is closed:
Fix
. Take affine transform on
, so that
.
Take some
, such that
. Then consider the "radial-directional" derivative of
on the Euclidean sphere
.
for all
.
Since
is compact, it achieves minimal value
at some
.
Since
is strictly convex,
. Then
.
Since
is
in
,
is continuous in
, thus
is closed if
is.
* Projection
is well-defined when
is closed and convex.
Fix
. Take some
, then let
. Then draw the Bregman ball
. It is closed and bounded, thus compact. Since
is continuous and strictly convex on it, and bounded below by
, it achieves a unique minimum on it.
* Pythagorean inequality.
By cosine law,
, which must be
, since
minimizes
in
, and
is convex.
* Pythagorean equality when
is in the relative interior of
.
If
, then since
is in the relative interior, we can move from
in the direction opposite of
, to decrease
, contradiction.
Thus
.
Classification theorems
* The only symmetric Bregman divergences on
are squared generalized Euclidean distances (
Mahalanobis distance The Mahalanobis distance is a measure of the distance between a point ''P'' and a distribution ''D'', introduced by P. C. Mahalanobis in 1936. Mahalanobis's definition was prompted by the problem of identifying the similarities of skulls based ...
), that is,
for some
positive definite In mathematics, positive definiteness is a property of any object to which a bilinear form or a sesquilinear form may be naturally associated, which is positive-definite. See, in particular:
* Positive-definite bilinear form
* Positive-definite ...
.
The following two characterizations are for divergences on
, the set of all probability measures on
, with
.
Define a divergence on
as any function of type