
In
image processing
An image is a visual representation of something. It can be two-dimensional, three-dimensional, or somehow otherwise feed into the visual system to convey information. An image can be an artifact, such as a photograph or other two-dimensiona ...
, histogram matching or histogram specification is the transformation of an image so that its
histogram
A histogram is an approximate representation of the distribution of numerical data. The term was first introduced by Karl Pearson. To construct a histogram, the first step is to " bin" (or "bucket") the range of values—that is, divide the ent ...
matches a specified histogram. The well-known
histogram equalization method is a special case in which the specified histogram is
uniformly distributed.
It is possible to use histogram matching to balance detector responses as a relative detector calibration technique. It can be used to normalize two images, when the images were acquired at the same local illumination (such as shadows) over the same location, but by different sensors, atmospheric conditions or global illumination.
Implementation
Consider a grayscale input image X. It has a probability density function p
r(r), where r is a grayscale value, and p
r(r) is the probability of that value. This probability can easily be computed from the histogram of the image by
Where n
j is the frequency of the grayscale value r
j, and n is the total number of pixels in the image.
Now consider a desired output probability density function p
z(z). A transformation of p
r(r) is needed to convert it to p
z(z).
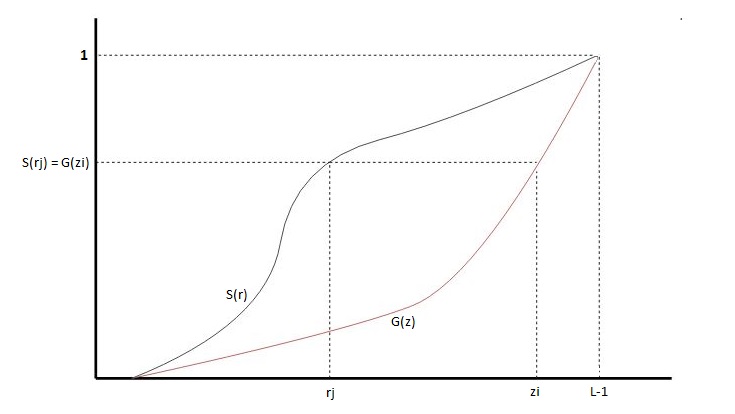
Each pdf (probability density function) can easily be mapped to its cumulative distribution function by
:
:
Where L is the total number of gray level (256 for a standard image).
The idea is to map each r value in X to the z value that has the same probability in the desired
pdf
Portable Document Format (PDF), standardized as ISO 32000, is a file format developed by Adobe in 1992 to present documents, including text formatting and images, in a manner independent of application software, hardware, and operating systems. ...
. I.e. ''S''(''r''
''j'') = ''G''(''z''
''i'') or ''z'' = ''G''
−1(''S''(''r'')).
Example
The following input grayscale image is to be changed to match the reference histogram.
The input image has the following histogram

It will be matched to this reference histogram to emphasize the lower gray levels.

After matching, the output image has the following histogram

And looks like this
Algorithm
Given two images, the reference and the target images, we compute their histograms. Following, we calculate the
cumulative distribution function
In probability theory and statistics, the cumulative distribution function (CDF) of a real-valued random variable X, or just distribution function of X, evaluated at x, is the probability that X will take a value less than or equal to x.
Ev ...
s of the two images' histograms –
for the reference image and
for the target image. Then for each gray level