
Cross-validation, sometimes called rotation estimation
or out-of-sample testing, is any of various similar
model validation techniques for assessing how the results of a
statistical
Statistics (from German: ''Statistik'', "description of a state, a country") is the discipline that concerns the collection, organization, analysis, interpretation, and presentation of data. In applying statistics to a scientific, industria ...
analysis will
generalize
A generalization is a form of abstraction whereby common properties of specific instances are formulated as general concepts or claims. Generalizations posit the existence of a domain or set of elements, as well as one or more common characteri ...
to an independent data set.
Cross-validation is a
resampling method that uses different portions of the data to test and train a model on different iterations. It is mainly used in settings where the goal is prediction, and one wants to estimate how
accurately a
predictive model will perform in practice. In a prediction problem, a model is usually given a dataset of ''known data'' on which training is run (''training dataset''), and a dataset of ''unknown data'' (or ''first seen'' data) against which the model is tested (called the
validation dataset or ''testing set'').
The goal of cross-validation is to test the model's ability to predict new data that was not used in estimating it, in order to flag problems like
overfitting
mathematical modeling, overfitting is "the production of an analysis that corresponds too closely or exactly to a particular set of data, and may therefore fail to fit to additional data or predict future observations reliably". An overfitt ...
or
selection bias
Selection bias is the bias introduced by the selection of individuals, groups, or data for analysis in such a way that proper randomization is not achieved, thereby failing to ensure that the sample obtained is representative of the population int ...
and to give an insight on how the model will generalize to an independent dataset (i.e., an unknown dataset, for instance from a real problem).
One round of cross-validation involves
partitioning a
sample of
data into
complementary subsets, performing the analysis on one subset (called the ''training set''), and validating the analysis on the other subset (called the ''validation set'' or ''testing set''). To reduce
variability, in most methods multiple rounds of cross-validation are performed using different partitions, and the validation results are combined (e.g. averaged) over the rounds to give an estimate of the model's predictive performance.
In summary, cross-validation combines (averages) measures of
fitness in prediction to derive a more accurate estimate of model prediction performance.
Motivation
Assume a
model with one or more unknown
parameters, and a data set to which the model can be fit (the training data set). The fitting process
optimizes the model parameters to make the model fit the training data as well as possible. If an
independent sample of validation data is taken from the same
population as the training data, it will generally turn out that the model does not fit the validation data as well as it fits the training data. The size of this difference is likely to be large especially when the size of the training data set is small, or when the number of parameters in the model is large. Cross-validation is a way to estimate the size of this effect.
In linear regression, there exist
real ''response values'' ''y''
1, ..., ''y
n'', and ''n'' ''p''-dimensional
vector ''covariates'' ''x''
1, ..., ''x''
''n''. The components of the vector ''x''
''i'' are denoted ''x''
''i''1, ..., ''x''
''ip''. If
least squares
The method of least squares is a standard approach in regression analysis to approximate the solution of overdetermined systems (sets of equations in which there are more equations than unknowns) by minimizing the sum of the squares of the res ...
is used to fit a function in the form of a
hyperplane
In geometry, a hyperplane is a subspace whose dimension is one less than that of its ''ambient space''. For example, if a space is 3-dimensional then its hyperplanes are the 2-dimensional planes, while if the space is 2-dimensional, its hyper ...
''ŷ'' = ''a'' + ''β''
T''x'' to the data (''x''
''i'', ''y''
''i'')
1 ≤ ''i'' ≤ ''n'', then the fit can be assessed using the
mean squared error (MSE). The MSE for given estimated parameter values ''a'' and ''β'' on the training set (''x''
''i'', ''y''
''i'')
1 ≤ ''i'' ≤ ''n'' is defined as:
:
If the model is correctly specified, it can be shown under mild assumptions that the
expected value
In probability theory, the expected value (also called expectation, expectancy, mathematical expectation, mean, average, or first moment) is a generalization of the weighted average. Informally, the expected value is the arithmetic mean of a l ...
of the MSE for the training set is (''n'' − ''p'' − 1)/(''n'' + ''p'' + 1) < 1 times the expected value of the MSE for the validation set (the expected value is taken over the distribution of training sets). Thus, a fitted model and computed MSE on the training set will result in an optimistically
biased assessment of how well the model will fit an independent data set. This biased estimate is called the ''in-sample'' estimate of the fit, whereas the cross-validation estimate is an ''out-of-sample'' estimate.
Since in linear regression it is possible to directly compute the factor (''n'' − ''p'' − 1)/(''n'' + ''p'' + 1) by which the training MSE underestimates the validation MSE under the assumption that the model specification is valid, cross-validation can be used for checking whether the model has been
overfitted, in which case the MSE in the validation set will substantially exceed its anticipated value. (Cross-validation in the context of linear regression is also useful in that it can be used to select an optimally
regularized cost function.)
In most other regression procedures (e.g.
logistic regression), there is no simple formula to compute the expected out-of-sample fit. Cross-validation is, thus, a generally applicable way to predict the performance of a model on unavailable data using numerical computation in place of theoretical analysis.
Types
Two types of cross-validation can be distinguished: exhaustive and non-exhaustive cross-validation.
Exhaustive cross-validation
Exhaustive cross-validation methods are cross-validation methods which learn and test on all possible ways to divide the original sample into a training and a validation set.
Leave-p-out cross-validation
Leave-''p''-out cross-validation (LpO CV) involves using ''p'' observations as the validation set and the remaining observations as the training set. This is repeated on all ways to cut the original sample on a validation set of ''p'' observations and a training set.
LpO cross-validation require training and validating the model
times, where ''n'' is the number of observations in the original sample, and where
is the
binomial coefficient
In mathematics, the binomial coefficients are the positive integers that occur as coefficients in the binomial theorem. Commonly, a binomial coefficient is indexed by a pair of integers and is written \tbinom. It is the coefficient of the t ...
. For ''p'' > 1 and for even moderately large ''n'', LpO CV can become computationally infeasible. For example, with ''n'' = 100 and ''p'' = 30,
A variant of LpO cross-validation with p=2 known as leave-pair-out cross-validation has been recommended as a nearly unbiased method for estimating the area under
ROC curve of binary classifiers.
Leave-one-out cross-validation
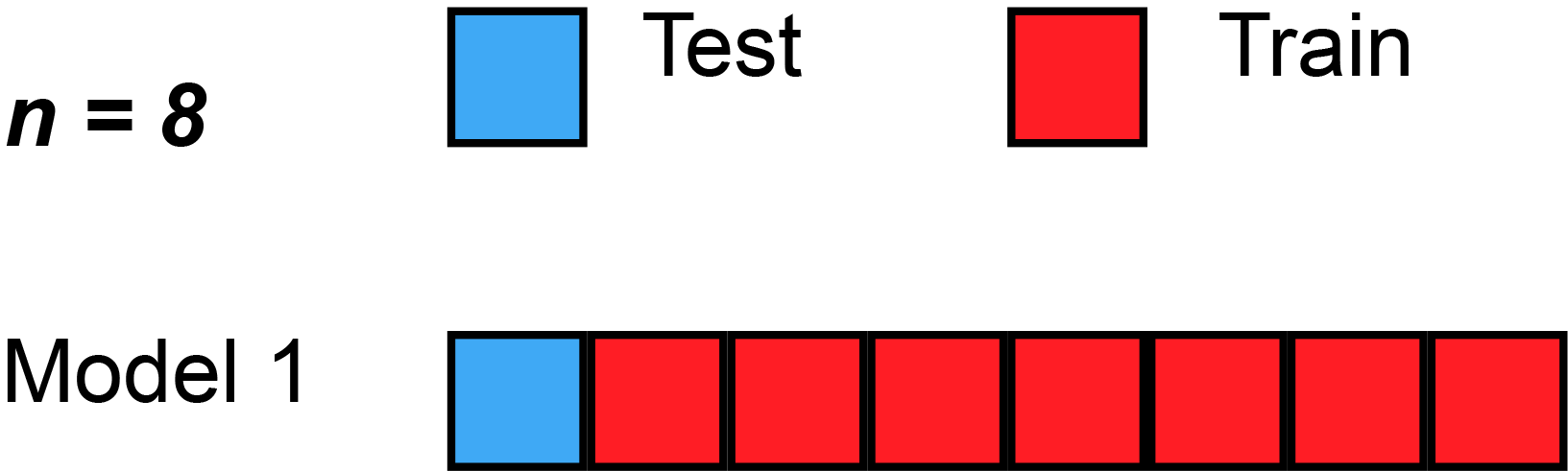
Leave-''one''-out cross-validation (LOOCV) is a particular case of leave-''p''-out cross-validation with ''p'' = 1.The process looks similar to
jackknife; however, with cross-validation one computes a statistic on the left-out sample(s), while with jackknifing one computes a statistic from the kept samples only.
LOO cross-validation requires less computation time than LpO cross-validation because there are only
passes rather than
. However,
passes may still require quite a large computation time, in which case other approaches such as k-fold cross validation may be more appropriate.
Pseudo-code algorithm:
Input:
x,
y,
interpolate( x_in, y_in, x_out ),
Output:
err,
Steps:
err ← 0
for i ← 1, ..., N do
// define the cross-validation subsets
x_in ← (x
..., x
− 1 x
+ 1 ..., x
y_in ← (y
..., y
− 1 y
+ 1 ..., y
x_out ← x
y_out ← interpolate(x_in, y_in, x_out)
err ← err + (y
− y_out)^2
end for
err ← err/N
Non-exhaustive cross-validation
Non-exhaustive cross validation methods do not compute all ways of splitting the original sample. These methods are approximations of leave-''p''-out cross-validation.
''k''-fold cross-validation

In ''k''-fold cross-validation, the original sample is randomly partitioned into ''k'' equal sized subsamples. Of the ''k'' subsamples, a single subsample is retained as the validation data for testing the model, and the remaining ''k'' − 1 subsamples are used as training data. The cross-validation process is then repeated ''k'' times, with each of the ''k'' subsamples used exactly once as the validation data. The ''k'' results can then be averaged to produce a single estimation. The advantage of this method over repeated random sub-sampling (see below) is that all observations are used for both training and validation, and each observation is used for validation exactly once. 10-fold cross-validation is commonly used,
but in general ''k'' remains an unfixed parameter.
For example, setting ''k'' = ''2'' results in 2-fold cross-validation. In 2-fold cross-validation, we randomly shuffle the dataset into two sets ''d''
0 and ''d''
1, so that both sets are equal size (this is usually implemented by shuffling the data array and then splitting it in two). We then train on ''d''
0 and validate on ''d''
1, followed by training on ''d''
1 and validating on ''d''
0.
When ''k'' = ''n'' (the number of observations), ''k''-fold cross-validation is equivalent to leave-one-out cross-validation.
In ''stratified'' ''k''-fold cross-validation, the partitions are selected so that the mean response value is approximately equal in all the partitions. In the case of binary classification, this means that each partition contains roughly the same proportions of the two types of class labels.
In ''repeated'' cross-validation the data is randomly split into ''k'' partitions several times. The performance of the model can thereby be averaged over several runs, but this is rarely desirable in practice.
Holdout method
In the holdout method, we randomly assign data points to two sets ''d''
0 and ''d''
1, usually called the training set and the test set, respectively. The size of each of the sets is arbitrary although typically the test set is smaller than the training set. We then train (build a model) on ''d''
0 and test (evaluate its performance) on ''d''
1.
In typical cross-validation, results of multiple runs of model-testing are averaged together; in contrast, the holdout method, in isolation, involves a single run. It should be used with caution because without such averaging of multiple runs, one may achieve highly misleading results. One's indicator of predictive accuracy (
''F''*) will tend to be unstable since it will not be smoothed out by multiple iterations (see below). Similarly, indicators of the specific role played by various predictor variables (e.g., values of regression coefficients) will tend to be unstable.
While the holdout method can be framed as "the simplest kind of cross-validation", many sources instead classify holdout as a type of simple validation, rather than a simple or degenerate form of cross-validation.
Repeated random sub-sampling validation
This method, also known as
Monte Carlo cross-validation,
creates multiple random splits of the dataset into training and validation data. For each such split, the model is fit to the training data, and predictive accuracy is assessed using the validation data. The results are then averaged over the splits. The advantage of this method (over ''k''-fold cross validation) is that the proportion of the training/validation split is not dependent on the number of iterations (i.e., the number of partitions). The disadvantage of this method is that some observations may never be selected in the validation subsample, whereas others may be selected more than once. In other words, validation subsets may overlap. This method also exhibits
Monte Carlo variation, meaning that the results will vary if the analysis is repeated with different random splits.
As the number of random splits approaches infinity, the result of repeated random sub-sampling validation tends towards that of leave-p-out cross-validation.
In a stratified variant of this approach, the random samples are generated in such a way that the mean response value (i.e. the dependent variable in the regression) is equal in the training and testing sets. This is particularly useful if the responses are
dichotomous with an unbalanced representation of the two response values in the data.
A method which applies repeated random sub-sampling is
RANSAC.
Nested cross-validation
When cross-validation is used simultaneously for selection of the best set of
hyperparameters
In Bayesian statistics, a hyperparameter is a parameter of a prior distribution; the term is used to distinguish them from parameters of the model for the underlying system under analysis.
For example, if one is using a beta distribution to mo ...
and for error estimation (and assessment of generalization capacity), a nested cross-validation is required. Many variants exist. At least two variants can be distinguished:
k*l-fold cross-validation
This is a truly nested variant which contains an outer loop of ''k'' sets and an inner loop of ''l'' sets. The total data set is split into ''k'' sets. One by one, a set is selected as the (outer) test set and the ''k'' - 1 other sets are combined into the corresponding outer training set. This is repeated for each of the ''k'' sets. Each outer training set is further sub-divided into ''l'' sets. One by one, a set is selected as inner test (validation) set and the ''l'' - 1 other sets are combined into the corresponding inner training set. This is repeated for each of the ''l'' sets. The inner training sets are used to fit model parameters, while the outer test set is used as a validation set to provide an unbiased evaluation of the model fit. Typically, this is repeated for many different hyperparameters (or even different model types) and the validation set is used to determine the best hyperparameter set (and model type) for this inner training set. After this, a new model is fit on the entire outer training set, using the best set of hyperparameters from the inner cross-validation. The performance of this model is then evaluated using the outer test set.
k-fold cross-validation with validation and test set
This is a type of k*l-fold cross-validation when ''l'' = ''k'' - 1. A single k-fold cross-validation is used with both a
validation and test set. The total data set is split into ''k'' sets. One by one, a set is selected as test set. Then, one by one, one of the remaining sets is used as a validation set and the other ''k'' - 2 sets are used as training sets until all possible combinations have been evaluated. Similar to the k*l-fold cross validation, the training set is used for model fitting and the validation set is used for model evaluation for each of the hyperparameter sets. Finally, for the selected parameter set, the test set is used to evaluate the model with the best parameter set. Here, two variants are possible: either evaluating the model that was trained on the training set or evaluating a new model that was fit on the combination of the training and the validation set.
Measures of fit
The goal of cross-validation is to estimate the expected level of fit of a model to a data set that is independent of the data that were used to train the model. It can be used to estimate any quantitative measure of fit that is appropriate for the data and model. For example, for
binary classification problems, each case in the validation set is either predicted correctly or incorrectly. In this situation the misclassification error rate can be used to summarize the fit, although other measures like
positive predictive value
The positive and negative predictive values (PPV and NPV respectively) are the proportions of positive and negative results in statistics and diagnostic tests that are true positive and true negative results, respectively. The PPV and NPV descr ...
could also be used. When the value being predicted is continuously distributed, the
mean squared error,
root mean squared error or
median absolute deviation could be used to summarize the errors.
Using prior information
When users apply cross-validation to select a good configuration
, then they might want to balance the cross-validated choice with their own estimate of the configuration. In this way, they can attempt to counter the volatility of cross-validation when the sample size is small and include relevant information from previous research. In a forecasting combination exercise, for instance, cross-validation can be applied to estimate the weights that are assigned to each forecast. Since a simple equal-weighted forecast is difficult to beat, a penalty can be added for deviating from equal weights.
Or, if cross-validation is applied to assign individual weights to observations, then one can penalize deviations from equal weights to avoid wasting potentially relevant information.
Hoornweg (2018) shows how a tuning parameter
can be defined so that a user can intuitively balance between the accuracy of cross-validation and the simplicity of sticking to a reference parameter
that is defined by the user.
If
denotes the
candidate configuration that might be selected, then the
loss function
In mathematical optimization and decision theory, a loss function or cost function (sometimes also called an error function) is a function that maps an event or values of one or more variables onto a real number intuitively representing some "cost ...
that is to be minimized can be defined as
:
Relative accuracy can be quantified as
, so that the mean squared error of a candidate
is made relative to that of a user-specified
. The relative simplicity term measures the amount that
deviates from
relative to the maximum amount of deviation from
. Accordingly, relative simplicity can be specified as
, where
corresponds to the
value with the highest permissible deviation from
. With