Features from accelerated segment test (FAST) is a
corner detection
Corner detection is an approach used within computer vision systems to extract certain kinds of features and infer the contents of an image. Corner detection is frequently used in motion detection, image registration, video tracking, image mo ...
method, which could be used to extract
feature
Feature may refer to:
Computing
* Feature (CAD), could be a hole, pocket, or notch
* Feature (computer vision), could be an edge, corner or blob
* Feature (software design) is an intentional distinguishing characteristic of a software item ...
points and later used to track and map objects in many
computer vision
Computer vision is an Interdisciplinarity, interdisciplinary scientific field that deals with how computers can gain high-level understanding from digital images or videos. From the perspective of engineering, it seeks to understand and automate t ...
tasks. The FAST corner detector was originally developed by Edward Rosten and Tom Drummond, and was published in 2006. The most promising advantage of the FAST corner detector is its computational efficiency. Referring to its name, it is indeed faster than many other well-known feature extraction methods, such as
difference of Gaussians In imaging science, difference of Gaussians (DoG) is a feature enhancement algorithm that involves the subtraction of one Gaussian blurred version of an original image from another, less blurred version of the original. In the simple case of grays ...
(DoG) used by the
SIFT
A sieve, fine mesh strainer, or sift, is a device for separating wanted elements from unwanted material or for controlling the particle size distribution of a sample, using a screen such as a woven mesh or net or perforated sheet materia ...
,
SUSAN
Susan is a feminine given name, from Persian "Susan" (lily flower), from Egyptian '' sšn'' and Coptic ''shoshen'' meaning "lotus flower", from Hebrew ''Shoshana'' meaning "lily" (in modern Hebrew this also means "rose" and a flower in general), ...
and
Harris
Harris may refer to:
Places Canada
* Harris, Ontario
* Northland Pyrite Mine (also known as Harris Mine)
* Harris, Saskatchewan
* Rural Municipality of Harris No. 316, Saskatchewan
Scotland
* Harris, Outer Hebrides (sometimes called the Isle o ...
detectors. Moreover, when machine learning techniques are applied, superior performance in terms of computation time and resources can be realised. The FAST corner detector is very suitable for real-time video processing application because of this high-speed performance.
Segment test detector
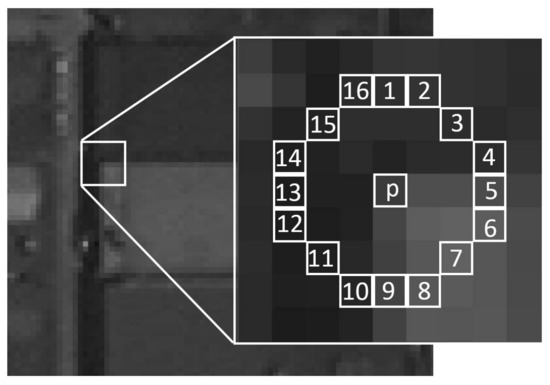
FAST corner detector uses a circle of 16 pixels (a
Bresenham circle of radius 3) to classify whether a candidate point p is actually a corner. Each pixel in the circle is labeled from integer number 1 to 16 clockwise. If a set of N contiguous pixels in the circle are all brighter than the intensity of candidate pixel p (denoted by I
p) plus a threshold value t or all darker than the intensity of candidate pixel p minus threshold value t, then p is classified as corner. The conditions can be written as:
*Condition 1: A set of N contiguous pixels S,
, the intensity of x > I
p + threshold, or
*Condition 2: A set of N contiguous pixels S,
,
So when either of the two conditions is met, candidate p can be classified as a corner. There is a tradeoff of choosing N, the number of contiguous pixels and the threshold value t. On one hand the number of detected corner points should not be too many, on the other hand, the high performance should not be achieved by sacrificing computational efficiency. Without the improvement of
machine learning
Machine learning (ML) is a field of inquiry devoted to understanding and building methods that 'learn', that is, methods that leverage data to improve performance on some set of tasks. It is seen as a part of artificial intelligence.
Machine ...
, N is usually chosen as 12. A high-speed test method could be applied to exclude non-corner points.
High-speed test
The high-speed test for rejecting non-corner points is operated by examining 4 example pixels, namely pixel 1, 9, 5 and 13. Because there should be at least 12 contiguous pixels that are whether all brighter or darker than the candidate corner, so there should be at least 3 pixels out of these 4 example pixels that are all brighter or darker than the candidate corner. Firstly pixels 1 and 9 are examined, if both I
1 and I
9 are within
p - t, Ip + t">p - t, Ip + t then candidate p is not a corner. Otherwise pixels 5 and 13 are further examined to check whether three of them are brighter than I
p + t or darker than I
p - t. If there exists 3 of them that are either brighter or darker, the rest pixels are then examined for final conclusion. And according to the inventor in his first paper, on average 3.8 pixels are needed to check for candidate corner pixel. Compared with 8.5 pixels for each candidate corner, 3.8 is really a great reduction which could highly improve the performance.
However, there are several weaknesses for this test method:
# The high-speed test cannot be generalized well for N < 12. If N < 12, it would be possible that a candidate p is a corner and only 2 out of 4 example test pixels are both brighter I
p + t or darker than I
p - t.
# The efficiency of the detector depends on the choice and ordering of these selected test pixels. However it is unlikely that the chosen pixels are optimal which take concerns about the distribution of corner appearances.
# Multiple features are detected adjacent to one another
Improvement with machine learning
In order to address the first two weakness points of high-speed test, a
machine learning
Machine learning (ML) is a field of inquiry devoted to understanding and building methods that 'learn', that is, methods that leverage data to improve performance on some set of tasks. It is seen as a part of artificial intelligence.
Machine ...
approach is introduced to help improve the detecting algorithm. This machine learning approach operates in two stages. Firstly, corner detection with a given N is processed on a set of training images which are preferable from the target application domain. Corners are detected through the simplest implementation which literally extracts a ring of 16 pixels and compares the intensity values with an appropriate threshold.
For candidate p, each location on the circle x ∈ can be denoted by p→x. The state of each pixel, S
p→x must be in one of the following three states:
* d, I
p→x ≤ I
p - t (darker)
* s, I
p - t ≤ I
p→x ≤ I
p + t (similar)
* b, I
p→x≥ I
p + t (brighter)
Then choosing an x (same for all p) partitions P (the set of all pixels of all training images) into 3 different subsets, P
d, P
s, P
b where:
* P
d =
* P
s =
* P
b =
Secondly, a
decision tree
A decision tree is a decision support tool that uses a tree-like model of decisions and their possible consequences, including chance event outcomes, resource costs, and utility. It is one way to display an algorithm that only contains co ...
algorithm, the
ID3 algorithm
In decision tree learning, ID3 (Iterative Dichotomiser 3) is an algorithm invented by Ross QuinlanQuinlan, J. R. 1986. Induction of Decision Trees. Mach. Learn. 1, 1 (Mar. 1986), 81–106 used to generate a decision tree from a dataset. ID3 is the ...
is applied to the 16 locations in order to achieve the maximum
information gain
Information is an abstract concept that refers to that which has the power to inform. At the most fundamental level information pertains to the interpretation of that which may be sensed. Any natural process that is not completely random, ...
. Let K
p be a boolean variable which indicates whether p is a corner, then the
entropy
Entropy is a scientific concept, as well as a measurable physical property, that is most commonly associated with a state of disorder, randomness, or uncertainty. The term and the concept are used in diverse fields, from classical thermodyna ...
of K
p is used to measure the information of p being a corner. For a set of pixels Q, the total entropy of K
Q (not normalized) is:
*H(Q) = ( c + n ) log
2( c + n ) - clog
2c - nlog
2n
** where c = , , (number of corners)
** where n = , , (number of non-corners)
The
information gain
Information is an abstract concept that refers to that which has the power to inform. At the most fundamental level information pertains to the interpretation of that which may be sensed. Any natural process that is not completely random, ...
can then be represented as:
*H
g= H(P) - H(P
b) - H(P
s) - H(P
d)
A recursive process is applied to each subsets in order to select each x that could maximize the information gain. For example, at first an x is selected to partition P into P
d, P
s, P
b with the most information; then for each subset P
d, P
s, P
b, another y is selected to yield the most information gain (notice that the y could be the same as x ). This recursive process ends when the entropy is zero so that either all pixels in that subset are corners or non-corners.
This generated
decision tree
A decision tree is a decision support tool that uses a tree-like model of decisions and their possible consequences, including chance event outcomes, resource costs, and utility. It is one way to display an algorithm that only contains co ...
can then be converted into programming code, such as
C and
C++, which is just a bunch of nested if-else statements. For optimization purpose,
profile-guided optimization
Profile-guided optimization (PGO, sometimes pronounced as ''pogo''), also known as profile-directed feedback (PDF), and feedback-directed optimization (FDO) is a compiler optimization technique in computer programming that uses profiling to impr ...
is used to compile the code. The complied code is used as corner detector later for other images.
Notice that the corners detected using this decision tree algorithm should be slightly different from the results using segment test detector. This is because that
decision tree model
In computational complexity the decision tree model is the model of computation in which an algorithm is considered to be basically a decision tree, i.e., a sequence of ''queries'' or ''tests'' that are done adaptively, so the outcome of the prev ...
depends on the training data, which could not cover all possible corners.
Non-maximum suppression
"Since the segment test does not compute a corner response function,
non-maximum suppression can not be applied directly to the resulting features." However, if N is fixed, for each pixel p the corner strength is defined as the maximum value of t that makes p a corner. Two approaches therefore could be used:
*A
binary search algorithm
In computer science, binary search, also known as half-interval search, logarithmic search, or binary chop, is a search algorithm that finds the position of a target value within a sorted array. Binary search compares the target value to the ...
could be applied to find the biggest t for which p is still a corner. So each time a different t is set for the decision tree algorithm. When it manages to find the biggest t, that t could be regarded as the corner strength.
*Another approach is an iteration scheme, where each time t is increased to the smallest value of which pass the test.
FAST-ER: Enhanced repeatability
FAST-ER detector is an improvement of the FAST detector using a
metaheuristic
In computer science and mathematical optimization, a metaheuristic is a higher-level procedure or heuristic designed to find, generate, or select a heuristic (partial search algorithm) that may provide a sufficiently good solution to an optimizatio ...
algorithm, in this case
simulated annealing
Simulated annealing (SA) is a probabilistic technique for approximating the global optimum of a given function. Specifically, it is a metaheuristic to approximate global optimization in a large search space for an optimization problem. ...
. So that after the optimization, the structure of the decision tree would be optimized and suitable for points with high repeatability. However, since
simulated annealing
Simulated annealing (SA) is a probabilistic technique for approximating the global optimum of a given function. Specifically, it is a metaheuristic to approximate global optimization in a large search space for an optimization problem. ...
is a metaheurisic algorithm, each time the algorithm would generate a different optimized decision tree. So it is better to take efficiently large amount of iterations to find a solution that is close to the real optimal. According to Rosten, it takes about 200 hours on a
Pentium 4
Pentium 4 is a series of single-core CPUs for desktops, laptops and entry-level servers manufactured by Intel. The processors were shipped from November 20, 2000 until August 8, 2008. The production of Netburst processors was active from 2000 ...
at 3 GHz which is 100 repeats of 100,000 iterations to optimize the FAST detector.
Comparison with other detectors
In Rosten's research,
[Edward Rosten]
FASTER and better: A machine learning approach to corner detection
/ref> FAST and FAST-ER detector are evaluated on several different datasets and compared with the DoG
The dog (''Canis familiaris'' or ''Canis lupus familiaris'') is a domesticated descendant of the wolf. Also called the domestic dog, it is derived from the extinct Pleistocene wolf, and the modern wolf is the dog's nearest living relativ ...
, Harris
Harris may refer to:
Places Canada
* Harris, Ontario
* Northland Pyrite Mine (also known as Harris Mine)
* Harris, Saskatchewan
* Rural Municipality of Harris No. 316, Saskatchewan
Scotland
* Harris, Outer Hebrides (sometimes called the Isle o ...
, Harris-Laplace, Shi-Tomasi, and SUSAN
Susan is a feminine given name, from Persian "Susan" (lily flower), from Egyptian '' sšn'' and Coptic ''shoshen'' meaning "lotus flower", from Hebrew ''Shoshana'' meaning "lily" (in modern Hebrew this also means "rose" and a flower in general), ...
corner detectors.
The parameter settings for the detectors (other than FAST) are as follows:
*Repeatability test result is presented as the averaged area under repeatability curves for 0-2000 corners per frame over all datasets (except the additive noise):
* Speed tests were performed on a 3.0 GHz Pentium 4-D computer. The dataset are divided into a training set and a test set. The training set consists of 101 monochrome
A monochrome or monochromatic image, object or palette is composed of one color (or values of one color). Images using only shades of grey are called grayscale (typically digital) or black-and-white (typically analog). In physics, monochr ...
images with a resolution of 992×668 pixels. The test set consists of 4968 frames of monochrome
A monochrome or monochromatic image, object or palette is composed of one color (or values of one color). Images using only shades of grey are called grayscale (typically digital) or black-and-white (typically analog). In physics, monochr ...
352×288 video. And the result is:
References
Bibliography
*
*
* {{cite book , last=Rosten , first=Edward , author2=Tom Drummond , title=Machine learning for high-speed corner detection , journal=European Conference on Computer Vision
, url=http://edwardrosten.com/work/rosten_2006_machine.pdf
, year=2006 , doi=10.1007/11744023_34 , volume=1, pages=430–443, series=Lecture Notes in Computer Science , isbn=978-3-540-33832-1 , citeseerx=10.1.1.64.8513
External links
Advanced Vision homepage
Feature detection (computer vision)